Revolutionizing Supply Chain Demand Forecasting in Pharma and Healthcare with AI
Navigating Modern Challenges in Pharma Supply Chains
In today’s fast-paced, technology-driven environment, pharmaceutical and healthcare supply chains face unprecedented challenges. Supply chain disruptions, rapidly evolving customer needs, and economic uncertainties—exacerbated by the pandemic—highlight the urgent need for accurate, tech-enabled demand forecasting. Traditional methods, heavily reliant on historical data, struggle to account for real-time changes in market conditions. AI and ML technologies are stepping in to transform supply chain operations, ensuring precision, efficiency, and scalability.
Client Overview
We partnered with a leading global pharmaceutical company managing:
- 5000+ SKUs spanning prescription and non-prescription drugs, vaccines, and cosmetics.
- 1000+ stocking depots, 10+ manufacturing facilities, and 100,000+ sales outlets.
- Distribution networks covering offline retail, e-commerce, institutional buyers, and modern retail.
The client sought to address complexities in supply chain forecasting with cutting-edge AI solutions.
Challenges in Pharma and Healthcare Supply Chains
- Dynamic Demand Shifts: Unpredictable consumption patterns driven by pandemics and health crises.
- Data Silos: Fragmented data from prescriptions, institutional orders, distribution, and sales channels.
- Stock Management: Striking the balance between overstocking and stockouts, impacting costs and patient satisfaction.
- Regulatory Pressures: Adhering to strict compliance standards while ensuring smooth operations.
- Channel-Specific Variability: Tailoring forecasts for diverse distribution channels like e-commerce and institutional supply chains.
AI-Powered Solution Architecture for Demand Forecasting
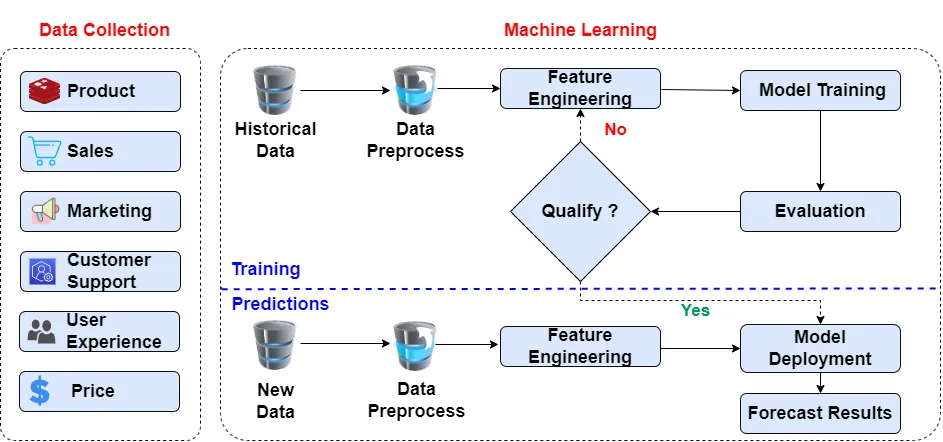
The Image shows the main stages of the solution architecture, including:
1. Data Collection and Preparation:
- Consolidated data from ERP, CRM, and POS systems.
- Incorporated external variables such as public health alerts, prescription trends, and seasonal factors.
- Standardized and cleaned datasets to ensure reliability.
2. Feature Engineering:
Derived pharma-specific features, including:
-
- Lag indicators for restocking cycles.
-
- Regional healthcare trends to predict localized demand.
-
- Expiry forecasts to minimize product wastage.
3. Model Selection and Training:
- Applied state-of-the-art models like XGBoost and LSTM to handle intricate demand patterns.
- Trained models on historical sales, distribution timelines, and purchasing behaviors.
4. Model Evaluation:
- Evaluated model performance using Mean Absolute Percentage Error (MAPE).
- Fine-tuned models to adapt to erratic demand for critical drugs and vaccines.
5. Deployment and Real-Time Monitoring:
- Deployed AI models into a centralized platform offering real-time forecasts.
- Enabled stakeholders to:
- Adjust production schedules dynamically.
- Optimize inventory and reduce overstock risks.
6. Outcome Visualization:
- Delivered interactive dashboards for:
- Tracking product-level trends.
- Simulating various discount strategies.
- Assessing supply chain disruptions.
As an example, assume that one needs to forecast the sales quantity of Product 1 for the next three months, specifically for Distributor A. So, to make a forecast, the following historical input data is available spanning the past four years.
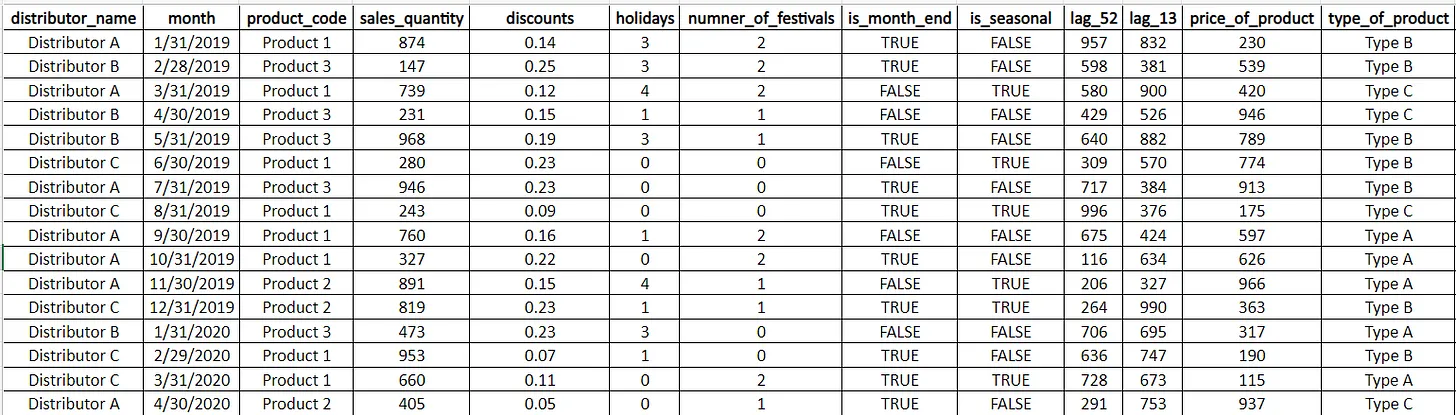
Upon completing the aforementioned steps outlined in the provided architecture, a set of methods have been tried to get the following results forecasted for Distributor A and Product 1
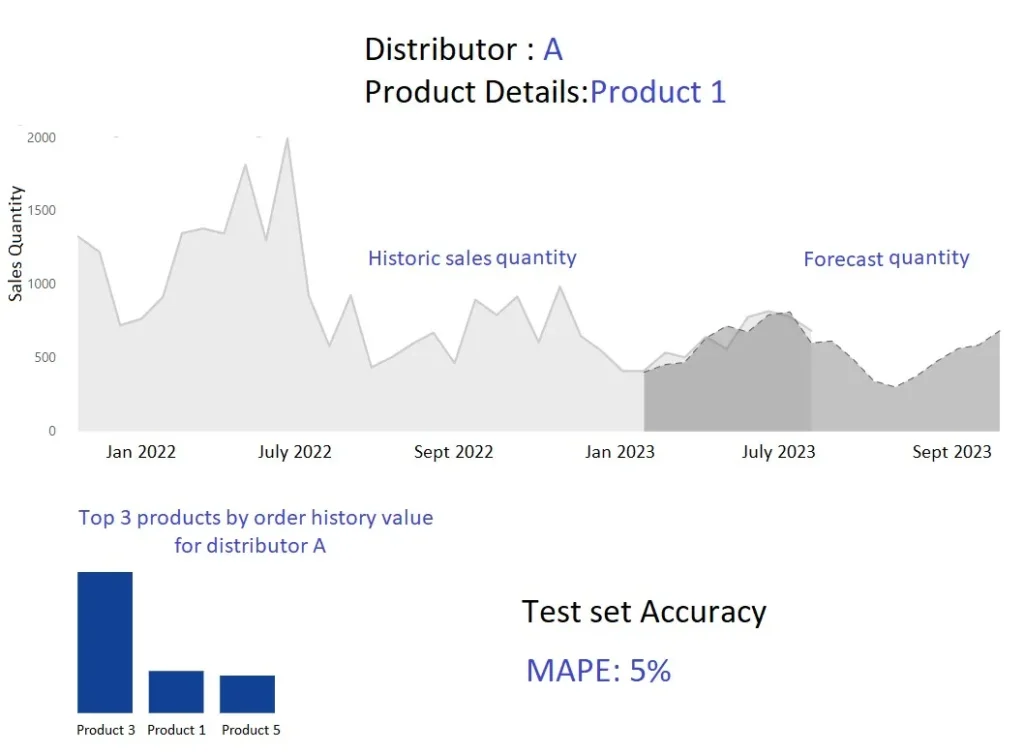
Transformative Impact of AI in Pharma Supply Chains
- Demand Sensing in Real Time: Monitors market trends and health crises to adjust forecasts.
- Automation: Reduces dependency on manual methods and eliminates biases.
- Enhanced Inventory Management: Cuts down on wastage and ensures availability.
- Scalability: Seamlessly handles global, multi-channel operations.
- Data-Driven Decisions: Empowers managers to respond proactively to challenges.
Measurable Benefits Delivered
- 98% Forecast Accuracy: Enhanced prediction for 5000+ SKUs.
- 30% Cost Reduction: Achieved through optimized inventory management.
- Rapid Adaptability: Faster response to market shifts during crises.
- Improved Patient Satisfaction: Ensured consistent availability of life-saving drugs.
Embracing AI for Pharma and Healthcare Supply Chains
AI-powered demand forecasting is no longer optional; it’s a necessity for organizations aiming to maintain a competitive edge. By leveraging advanced ML models and intelligent data platforms, pharmaceutical and healthcare businesses can:
- Respond swiftly to public health emergencies.
- Optimize production and reduce costs.
- Improve financial planning with accurate demand forecasts.
- Scale operations across complex, multi-regional supply chains.
Let’s Transform Together: Ready to explore how AI can optimize your supply chain operations? Connect with us to embark on this transformative journey.